Data-driven modeling and prediction of non-linearizable dynamics via spectral submanifolds
- PMID: 35169152
- PMCID: PMC8847615
- DOI: 10.1038/s41467-022-28518-y
Data-driven modeling and prediction of non-linearizable dynamics via spectral submanifolds
Abstract
We develop a methodology to construct low-dimensional predictive models from data sets representing essentially nonlinear (or non-linearizable) dynamical systems with a hyperbolic linear part that are subject to external forcing with finitely many frequencies. Our data-driven, sparse, nonlinear models are obtained as extended normal forms of the reduced dynamics on low-dimensional, attracting spectral submanifolds (SSMs) of the dynamical system. We illustrate the power of data-driven SSM reduction on high-dimensional numerical data sets and experimental measurements involving beam oscillations, vortex shedding and sloshing in a water tank. We find that SSM reduction trained on unforced data also predicts nonlinear response accurately under additional external forcing.
© 2022. The Author(s).
Conflict of interest statement
The authors declare no competing interests.
Figures
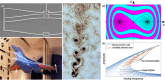
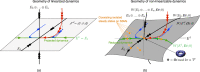
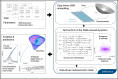
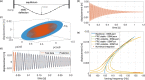
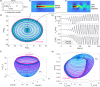
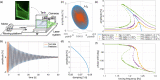
Similar articles
-
Data-driven modeling of subharmonic forced response due to nonlinear resonance.Sci Rep. 2024 Oct 29;14(1):25991. doi: 10.1038/s41598-024-77639-5. Sci Rep. 2024. PMID: 39472605 Free PMC article.
-
Data-driven nonlinear model reduction to spectral submanifolds in mechanical systems.Philos Trans A Math Phys Eng Sci. 2022 Aug 8;380(2229):20210194. doi: 10.1098/rsta.2021.0194. Epub 2022 Jun 20. Philos Trans A Math Phys Eng Sci. 2022. PMID: 35719078 Free PMC article.
-
Data-driven modeling and forecasting of chaotic dynamics on inertial manifolds constructed as spectral submanifolds.Chaos. 2024 Mar 1;34(3):033140. doi: 10.1063/5.0179741. Chaos. 2024. PMID: 38531092
-
The spike trains of inhibited pacemaker neurons seen through the magnifying glass of nonlinear analyses.Neuroscience. 1998 Dec;87(4):741-66. doi: 10.1016/s0306-4522(98)00086-4. Neuroscience. 1998. PMID: 9759964 Review.
-
Modeling of dynamical systems through deep learning.Biophys Rev. 2020 Nov 22;12(6):1311-20. doi: 10.1007/s12551-020-00776-4. Online ahead of print. Biophys Rev. 2020. PMID: 33222032 Free PMC article. Review.
Cited by
-
Data-driven modeling of subharmonic forced response due to nonlinear resonance.Sci Rep. 2024 Oct 29;14(1):25991. doi: 10.1038/s41598-024-77639-5. Sci Rep. 2024. PMID: 39472605 Free PMC article.
-
Data-driven linearization of dynamical systems.Nonlinear Dyn. 2024;112(21):18639-18663. doi: 10.1007/s11071-024-10026-x. Epub 2024 Aug 15. Nonlinear Dyn. 2024. PMID: 39219721 Free PMC article.
-
Learning the intrinsic dynamics of spatio-temporal processes through Latent Dynamics Networks.Nat Commun. 2024 Feb 28;15(1):1834. doi: 10.1038/s41467-024-45323-x. Nat Commun. 2024. PMID: 38418469 Free PMC article.
-
Data-driven nonlinear model reduction to spectral submanifolds in mechanical systems.Philos Trans A Math Phys Eng Sci. 2022 Aug 8;380(2229):20210194. doi: 10.1098/rsta.2021.0194. Epub 2022 Jun 20. Philos Trans A Math Phys Eng Sci. 2022. PMID: 35719078 Free PMC article.
References
-
- Holmes, P.J., Lumley, J.L., Berkooz, G., & Rowley, C.W. Turbulence, Coherent Structures, Dynamical Systems and Symmetry 2nd edn, (Cambridge Monographs on Mechanics. Cambridge University Press, 2012).
-
- Awrejcewicz, J., Krys’ko, V.A., & Vakakis, A.F. Order Reduction by Proper Orthogonal Decomposition (POD) Analysis, 279–320 (Springer, Berlin, Heidelberg, 2004).
-
- Lu K, et al. Review for order reduction based on proper orthogonal decomposition and outlooks of applications in mechanical systems. Mech. Sys. Signal Proc. 2019;123:264–297.
-
- Mohamed, K.S. Machine Learning for Model Order Reduction (Springer, Cham, 2018).
LinkOut - more resources
Full Text Sources