Sensor and Sensor Fusion Technology in Autonomous Vehicles: A Review
- PMID: 33803889
- PMCID: PMC8003231
- DOI: 10.3390/s21062140
Sensor and Sensor Fusion Technology in Autonomous Vehicles: A Review
Abstract
With the significant advancement of sensor and communication technology and the reliable application of obstacle detection techniques and algorithms, automated driving is becoming a pivotal technology that can revolutionize the future of transportation and mobility. Sensors are fundamental to the perception of vehicle surroundings in an automated driving system, and the use and performance of multiple integrated sensors can directly determine the safety and feasibility of automated driving vehicles. Sensor calibration is the foundation block of any autonomous system and its constituent sensors and must be performed correctly before sensor fusion and obstacle detection processes may be implemented. This paper evaluates the capabilities and the technical performance of sensors which are commonly employed in autonomous vehicles, primarily focusing on a large selection of vision cameras, LiDAR sensors, and radar sensors and the various conditions in which such sensors may operate in practice. We present an overview of the three primary categories of sensor calibration and review existing open-source calibration packages for multi-sensor calibration and their compatibility with numerous commercial sensors. We also summarize the three main approaches to sensor fusion and review current state-of-the-art multi-sensor fusion techniques and algorithms for object detection in autonomous driving applications. The current paper, therefore, provides an end-to-end review of the hardware and software methods required for sensor fusion object detection. We conclude by highlighting some of the challenges in the sensor fusion field and propose possible future research directions for automated driving systems.
Keywords: autonomous vehicles; calibration; camera; lidar; obstacle detection; perception; radar; self-driving cars; sensor fusion.
Conflict of interest statement
The authors declare no conflict of interest.
Figures
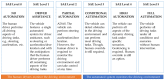
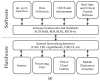
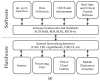
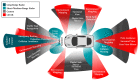
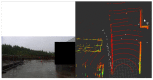
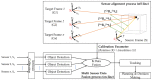
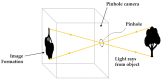
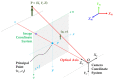
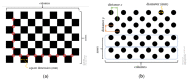
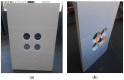
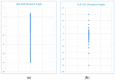
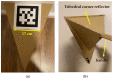
Similar articles
-
End-to-End Multimodal Sensor Dataset Collection Framework for Autonomous Vehicles.Sensors (Basel). 2023 Jul 29;23(15):6783. doi: 10.3390/s23156783. Sensors (Basel). 2023. PMID: 37571566 Free PMC article.
-
MmWave Radar and Vision Fusion for Object Detection in Autonomous Driving: A Review.Sensors (Basel). 2022 Mar 25;22(7):2542. doi: 10.3390/s22072542. Sensors (Basel). 2022. PMID: 35408157 Free PMC article. Review.
-
Sensor Fusion in Autonomous Vehicle with Traffic Surveillance Camera System: Detection, Localization, and AI Networking.Sensors (Basel). 2023 Mar 22;23(6):3335. doi: 10.3390/s23063335. Sensors (Basel). 2023. PMID: 36992043 Free PMC article.
-
Navigating an Automated Driving Vehicle via the Early Fusion of Multi-Modality.Sensors (Basel). 2022 Feb 13;22(4):1425. doi: 10.3390/s22041425. Sensors (Basel). 2022. PMID: 35214327 Free PMC article.
-
Deep Learning Sensor Fusion for Autonomous Vehicle Perception and Localization: A Review.Sensors (Basel). 2020 Jul 29;20(15):4220. doi: 10.3390/s20154220. Sensors (Basel). 2020. PMID: 32751275 Free PMC article. Review.
Cited by
-
A LiDAR-Camera Joint Calibration Algorithm Based on Deep Learning.Sensors (Basel). 2024 Sep 18;24(18):6033. doi: 10.3390/s24186033. Sensors (Basel). 2024. PMID: 39338778 Free PMC article.
-
A Survey on Sensor Failures in Autonomous Vehicles: Challenges and Solutions.Sensors (Basel). 2024 Aug 7;24(16):5108. doi: 10.3390/s24165108. Sensors (Basel). 2024. PMID: 39204805 Free PMC article. Review.
-
Extracting Vehicle Trajectories from Partially Overlapping Roadside Radar.Sensors (Basel). 2024 Jul 17;24(14):4640. doi: 10.3390/s24144640. Sensors (Basel). 2024. PMID: 39066038 Free PMC article.
-
A new two-step road extraction method in high resolution remote sensing images.PLoS One. 2024 Jul 18;19(7):e0305933. doi: 10.1371/journal.pone.0305933. eCollection 2024. PLoS One. 2024. PMID: 39024329 Free PMC article.
-
Multi-Tracking Sensor Architectures for Reconstructing Autonomous Vehicle Crashes: An Exploratory Study.Sensors (Basel). 2024 Jun 27;24(13):4194. doi: 10.3390/s24134194. Sensors (Basel). 2024. PMID: 39000972 Free PMC article.
References
-
- World Health Organization . Global Status Report on Road Safety. WHO; Geneva, Switzerland: 2018.
-
- Road | Mobility and Transport. [(accessed on 20 November 2020)]; Available online: https://ec.europa.eu/transport/themes/its/road_it.
-
- Autonomous Vehicle Market to Garner Growth 63.5% [(accessed on 19 November 2020)]; Available online: https://www.precedenceresearch.com/autonomous-vehicle-market.
-
- Glon R., Edelstein S. The History of Self-Driving Cars. [(accessed on 18 November 2020)];2020 Available online: https://www.digitaltrends.com/cars/history-of-self-driving-cars-milestones/
-
- Wiggers K. Waymo’s Autonomous Cars Have Driven 20 Million Miles on Public Roads. [(accessed on 18 November 2020)];2020 Available online: https://venturebeat.com/2020/01/06/waymos-autonomous-cars-have-driven-20...
Publication types
Grants and funding
LinkOut - more resources
Full Text Sources
Other Literature Sources