Prediction of breast cancer distant recurrence using natural language processing and knowledge-guided convolutional neural network
- PMID: 33250149
- PMCID: PMC7983067
- DOI: 10.1016/j.artmed.2020.101977
Prediction of breast cancer distant recurrence using natural language processing and knowledge-guided convolutional neural network
Abstract
Distant recurrence of breast cancer results in high lifetime risks and low 5-year survival rates. Early prediction of distant recurrent breast cancer could facilitate intervention and improve patients' life quality. In this study, we designed an EHR-based predictive model to estimate the distant recurrent probability of breast cancer patients. We studied the pathology reports and progress notes of 6,447 patients who were diagnosed with breast cancer at Northwestern Memorial Hospital between 2001 and 2015. Clinical notes were mapped to Concept unified identifiers (CUI) using natural language processing tools. Bag-of-words and pre-trained embedding were employed to vectorize words and CUI sequences. These features integrated with clinical features from structured data were downstreamed to conventional machine learning classifiers and Knowledge-guided Convolutional Neural Network (K-CNN). The best configuration of our model yielded an AUC of 0.888 and an F1-score of 0.5. Our work provides an automated method to predict breast cancer distant recurrence using natural language processing and deep learning approaches. We expect that through advanced feature engineering, better predictive performance could be achieved.
Keywords: Breast cancer; Distant recurrence; Entity embeddings; Knowledge-guided convolutional neural network; Word embeddings.
Copyright © 2020 Elsevier B.V. All rights reserved.
Figures
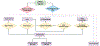
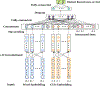
Similar articles
-
Using natural language processing and machine learning to identify breast cancer local recurrence.BMC Bioinformatics. 2018 Dec 28;19(Suppl 17):498. doi: 10.1186/s12859-018-2466-x. BMC Bioinformatics. 2018. PMID: 30591037 Free PMC article.
-
Medical subdomain classification of clinical notes using a machine learning-based natural language processing approach.BMC Med Inform Decis Mak. 2017 Dec 1;17(1):155. doi: 10.1186/s12911-017-0556-8. BMC Med Inform Decis Mak. 2017. PMID: 29191207 Free PMC article.
-
Predicting mortality in critically ill patients with diabetes using machine learning and clinical notes.BMC Med Inform Decis Mak. 2020 Dec 30;20(Suppl 11):295. doi: 10.1186/s12911-020-01318-4. BMC Med Inform Decis Mak. 2020. PMID: 33380338 Free PMC article.
-
A comparison of word embeddings for the biomedical natural language processing.J Biomed Inform. 2018 Nov;87:12-20. doi: 10.1016/j.jbi.2018.09.008. Epub 2018 Sep 12. J Biomed Inform. 2018. PMID: 30217670 Free PMC article.
-
Clinical text classification with rule-based features and knowledge-guided convolutional neural networks.BMC Med Inform Decis Mak. 2019 Apr 4;19(Suppl 3):71. doi: 10.1186/s12911-019-0781-4. BMC Med Inform Decis Mak. 2019. PMID: 30943960 Free PMC article.
Cited by
-
Using the Electronic Health Record to Develop a Gastric Cancer Risk Prediction Model.Gastro Hep Adv. 2024 Jul 14;3(7):910-916. doi: 10.1016/j.gastha.2024.07.001. eCollection 2024. Gastro Hep Adv. 2024. PMID: 39286619 Free PMC article.
-
Northwestern University resource and education development initiatives to advance collaborative artificial intelligence across the learning health system.Learn Health Syst. 2024 Apr 15;8(3):e10417. doi: 10.1002/lrh2.10417. eCollection 2024 Jul. Learn Health Syst. 2024. PMID: 39036530 Free PMC article.
-
Explanatory argumentation in natural language for correct and incorrect medical diagnoses.J Biomed Semantics. 2024 May 30;15(1):8. doi: 10.1186/s13326-024-00306-1. J Biomed Semantics. 2024. PMID: 38816758 Free PMC article.
-
Predicting which patients with cancer will see a psychiatrist or counsellor from their initial oncology consultation document using natural language processing.Commun Med (Lond). 2024 Apr 8;4(1):69. doi: 10.1038/s43856-024-00495-x. Commun Med (Lond). 2024. PMID: 38589545 Free PMC article.
-
Automatic Detection of Distant Metastasis Mentions in Radiology Reports in Spanish.JCO Clin Cancer Inform. 2024 Jan;8:e2300130. doi: 10.1200/CCI.23.00130. JCO Clin Cancer Inform. 2024. PMID: 38194615 Free PMC article.
References
-
- W. W.C. R. F. I. for Cancer Research), Diet, nutrition, physical activity and cancer: a global perspective. continuous update project expert report (2018).
-
- DeSantis C, Ma J, Bryan L, Jemal A, Breast cancer statistics, 2013, CA: a cancer journal for clinicians 64 (2014) 52–62. - PubMed
-
- DeSantis C, Siegel R, Bandi P, Jemal A, Breast cancer statistics, 2011, CA: a cancer journal for clinicians 61 (2011) 408–418. - PubMed
-
- Siegel RL, Miller KD, Jemal A, Cancer statistics, 2019, CA: a cancer journal for clinicians 69 (2019) 7–34. - PubMed
-
- Turner J, Hayes S, Reul-Hirche H, Improving the physical status and quality of life of women treated for breast cancer: a pilot study of a structured exercise intervention, Journal of surgical oncology 86 (2004) 141–146. - PubMed
MeSH terms
Grants and funding
LinkOut - more resources
Full Text Sources
Medical