Text Mining for Protein Docking
- PMID: 26650466
- PMCID: PMC4674139
- DOI: 10.1371/journal.pcbi.1004630
Text Mining for Protein Docking
Abstract
The rapidly growing amount of publicly available information from biomedical research is readily accessible on the Internet, providing a powerful resource for predictive biomolecular modeling. The accumulated data on experimentally determined structures transformed structure prediction of proteins and protein complexes. Instead of exploring the enormous search space, predictive tools can simply proceed to the solution based on similarity to the existing, previously determined structures. A similar major paradigm shift is emerging due to the rapidly expanding amount of information, other than experimentally determined structures, which still can be used as constraints in biomolecular structure prediction. Automated text mining has been widely used in recreating protein interaction networks, as well as in detecting small ligand binding sites on protein structures. Combining and expanding these two well-developed areas of research, we applied the text mining to structural modeling of protein-protein complexes (protein docking). Protein docking can be significantly improved when constraints on the docking mode are available. We developed a procedure that retrieves published abstracts on a specific protein-protein interaction and extracts information relevant to docking. The procedure was assessed on protein complexes from Dockground (http://dockground.compbio.ku.edu). The results show that correct information on binding residues can be extracted for about half of the complexes. The amount of irrelevant information was reduced by conceptual analysis of a subset of the retrieved abstracts, based on the bag-of-words (features) approach. Support Vector Machine models were trained and validated on the subset. The remaining abstracts were filtered by the best-performing models, which decreased the irrelevant information for ~ 25% complexes in the dataset. The extracted constraints were incorporated in the docking protocol and tested on the Dockground unbound benchmark set, significantly increasing the docking success rate.
Conflict of interest statement
The authors have declared that no competing interests exist.
Figures
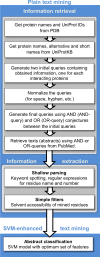
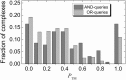
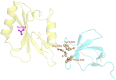
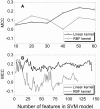
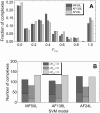
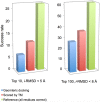
Similar articles
-
Natural language processing in text mining for structural modeling of protein complexes.BMC Bioinformatics. 2018 Mar 5;19(1):84. doi: 10.1186/s12859-018-2079-4. BMC Bioinformatics. 2018. PMID: 29506465 Free PMC article.
-
Simulated unbound structures for benchmarking of protein docking in the DOCKGROUND resource.BMC Bioinformatics. 2015 Jul 31;16(1):243. doi: 10.1186/s12859-015-0672-3. BMC Bioinformatics. 2015. PMID: 26227548 Free PMC article.
-
Dockground: A comprehensive data resource for modeling of protein complexes.Protein Sci. 2018 Jan;27(1):172-181. doi: 10.1002/pro.3295. Epub 2017 Oct 10. Protein Sci. 2018. PMID: 28891124 Free PMC article.
-
Protein-protein docking: from interaction to interactome.Biophys J. 2014 Oct 21;107(8):1785-1793. doi: 10.1016/j.bpj.2014.08.033. Biophys J. 2014. PMID: 25418159 Free PMC article. Review.
-
Rigid-Docking Approaches to Explore Protein-Protein Interaction Space.Adv Biochem Eng Biotechnol. 2017;160:33-55. doi: 10.1007/10_2016_41. Adv Biochem Eng Biotechnol. 2017. PMID: 27830312 Review.
Cited by
-
Review and Comparative Analysis of Methods and Advancements in Predicting Protein Complex Structure.Interdiscip Sci. 2024 Jun;16(2):261-288. doi: 10.1007/s12539-024-00626-x. Epub 2024 Jul 2. Interdiscip Sci. 2024. PMID: 38955920 Review.
-
Overview of methods for characterization and visualization of a protein-protein interaction network in a multi-omics integration context.Front Mol Biosci. 2022 Sep 8;9:962799. doi: 10.3389/fmolb.2022.962799. eCollection 2022. Front Mol Biosci. 2022. PMID: 36158572 Free PMC article. Review.
-
Natural product drug discovery in the artificial intelligence era.Chem Sci. 2021 Dec 13;13(6):1526-1546. doi: 10.1039/d1sc04471k. eCollection 2022 Feb 9. Chem Sci. 2021. PMID: 35282622 Free PMC article. Review.
-
Text mining for modeling of protein complexes enhanced by machine learning.Bioinformatics. 2021 May 1;37(4):497-505. doi: 10.1093/bioinformatics/btaa823. Bioinformatics. 2021. PMID: 32960948 Free PMC article.
-
Constructing knowledge graphs and their biomedical applications.Comput Struct Biotechnol J. 2020 Jun 2;18:1414-1428. doi: 10.1016/j.csbj.2020.05.017. eCollection 2020. Comput Struct Biotechnol J. 2020. PMID: 32637040 Free PMC article. Review.
References
-
- Sanchez R, Sali A. Advances in comparative protein-structure modeling. Curr Opin Struct Biol. 1997;7:206–14. - PubMed
-
- Aloy P, Ceulemans H, Stark A, Russell RB. The relationship between sequence and interaction divergence in proteins. J Mol Biol. 2003;332:989–98. - PubMed
-
- Lu L, Lu H, Skolnick J. MULTIPROSPECTOR: An algorithm for the prediction of protein-protein interactions by multimeric threading. Proteins. 2002;49:350–64. - PubMed
Grants and funding
LinkOut - more resources
Full Text Sources
Other Literature Sources