Mitochondrial variability as a source of extrinsic cellular noise
- PMID: 22412363
- PMCID: PMC3297557
- DOI: 10.1371/journal.pcbi.1002416
Mitochondrial variability as a source of extrinsic cellular noise
Abstract
We present a study investigating the role of mitochondrial variability in generating noise in eukaryotic cells. Noise in cellular physiology plays an important role in many fundamental cellular processes, including transcription, translation, stem cell differentiation and response to medication, but the specific random influences that affect these processes have yet to be clearly elucidated. Here we present a mechanism by which variability in mitochondrial volume and functionality, along with cell cycle dynamics, is linked to variability in transcription rate and hence has a profound effect on downstream cellular processes. Our model mechanism is supported by an appreciable volume of recent experimental evidence, and we present the results of several new experiments with which our model is also consistent. We find that noise due to mitochondrial variability can sometimes dominate over other extrinsic noise sources (such as cell cycle asynchronicity) and can significantly affect large-scale observable properties such as cell cycle length and gene expression levels. We also explore two recent regulatory network-based models for stem cell differentiation, and find that extrinsic noise in transcription rate causes appreciable variability in the behaviour of these model systems. These results suggest that mitochondrial and transcriptional variability may be an important mechanism influencing a large variety of cellular processes and properties.
Conflict of interest statement
The authors have declared that no competing interests exist.
Figures
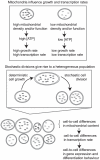
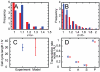


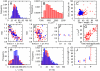










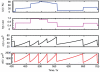




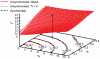








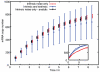



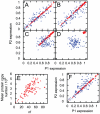


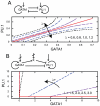

Similar articles
-
Pathway dynamics can delineate the sources of transcriptional noise in gene expression.Elife. 2021 Oct 12;10:e69324. doi: 10.7554/eLife.69324. Elife. 2021. PMID: 34636320 Free PMC article.
-
Quantifying intrinsic and extrinsic variability in stochastic gene expression models.PLoS One. 2013 Dec 31;8(12):e84301. doi: 10.1371/journal.pone.0084301. eCollection 2013. PLoS One. 2013. PMID: 24391934 Free PMC article.
-
Mitochondrial heterogeneity, metabolic scaling and cell death.Bioessays. 2017 Jul;39(7). doi: 10.1002/bies.201700001. Epub 2017 Jun 8. Bioessays. 2017. PMID: 28594445 Review.
-
Intercellular Variability in Protein Levels from Stochastic Expression and Noisy Cell Cycle Processes.PLoS Comput Biol. 2016 Aug 18;12(8):e1004972. doi: 10.1371/journal.pcbi.1004972. eCollection 2016 Aug. PLoS Comput Biol. 2016. PMID: 27536771 Free PMC article.
-
A role for mitochondrial aquaporins in cellular life-and-death decisions?Am J Physiol Cell Physiol. 2006 Aug;291(2):C195-202. doi: 10.1152/ajpcell.00641.2005. Epub 2006 Apr 19. Am J Physiol Cell Physiol. 2006. PMID: 16624989 Review.
Cited by
-
Mitochondrial protein heterogeneity stems from the stochastic nature of co-translational protein targeting in cell senescence.Nat Commun. 2024 Sep 27;15(1):8274. doi: 10.1038/s41467-024-52183-y. Nat Commun. 2024. PMID: 39333462 Free PMC article.
-
Stochastic Gene Expression in Proliferating Cells: Differing Noise Intensity in Single-Cell and Population Perspectives.bioRxiv [Preprint]. 2024 Jun 29:2024.06.28.601263. doi: 10.1101/2024.06.28.601263. bioRxiv. 2024. PMID: 38979195 Free PMC article. Preprint.
-
Gram-positive bacteria are primed for surviving lethal doses of antibiotics and chemical stress.bioRxiv [Preprint]. 2024 Jun 7:2024.05.28.596288. doi: 10.1101/2024.05.28.596288. bioRxiv. 2024. PMID: 38895422 Free PMC article. Preprint.
-
Feedback between stochastic gene networks and population dynamics enables cellular decision-making.Sci Adv. 2024 May 24;10(21):eadl4895. doi: 10.1126/sciadv.adl4895. Epub 2024 May 24. Sci Adv. 2024. PMID: 38787956 Free PMC article.
-
Significance of quantitative analyses of the impact of heterogeneity in mitochondrial content and shape on cell differentiation.Open Biol. 2024 Jan;14(1):230279. doi: 10.1098/rsob.230279. Epub 2024 Jan 17. Open Biol. 2024. PMID: 38228170 Free PMC article. Review.
References
Publication types
MeSH terms
LinkOut - more resources
Full Text Sources
Other Literature Sources