- 1Harvard University, Cambridge, MA, United States
- 2Perusall LLC, Brookline, MA, United States
In this paper, we illustrate the successful implementation of pre-class reading assignments through a social learning platform that allows students to discuss the reading online with their classmates. We show how the platform can be used to understand how students are reading before class. We find that, with this platform, students spend an above average amount of time reading (compared to that reported in the literature) and that most students complete their reading assignments before class. We identify specific reading behaviors that are predictive of in-class exam performance. We also demonstrate ways that the platform promotes active reading strategies and produces high-quality learning interactions between students outside class. Finally, we compare the exam performance of two cohorts of students, where the only difference between them is the use of the platform; we show that students do significantly better on exams when using the platform.
Introduction
Getting students to read the textbook before coming to class is an important problem in higher education. This is increasingly the case as more college classes are adopting “flipped” teaching strategies. A key principle of the flipped classroom model is that students benefit from having access to the instructor (and other peers) when working on activities that, in traditional classrooms, are typically done at home (like problem sets). Moving these activities in class improves student learning as it provides them the opportunity to actively engage with the instructor and each other (Herreid and Schiller, 2013). In a flipped class, the information transfer (traditionally accomplished by the instructor delivering a lecture during class) is moved outside the classroom to a pre-class assignment that students are expected to complete before coming to class. These pre-class assignments typically require students watch a video of a lecture online, or complete a reading. Moving the information delivery out of the classroom allows in-class time to be used for more interactive activities during which students can be actively engaged with instructors and other students.
When students are exposed to the material before class they are better able to follow material in class (Schwartz and Bransford, 1998), they ask more meaningful questions in class (Marcell, 2008), and they perform better on exams (Narloch et al., 2006; Dobson, 2008; Johnson and Kiviniemi, 2009). Students report that one of the most important factors in deciding whether to participate in class is reading the textbook beforehand (Karp and Yoels, 1976). The connection between pre-class reading and in-class participation is particularly relevant in flipped courses that rely on active in-class participation.
As pre-class reading assignments replace lectures in flipped courses and serve as the primary mechanism for information transfer, it is essential that students complete their assignments before class. Even in traditional (non-flipped) college courses, pre-class reading has been shown to be important for student learning and yet 60–80% of students do not read the textbook before coming to class (Cummings et al., 2002; Clump et al., 2004; Podolefsky and Finkelstein, 2006; Stelzer et al., 2009). Clump et al. (2004) studied the extent to which psychology students reported reading their textbooks and found that students only read, on average, 28% of the assigned reading before class and 70% before the exam.
Other studies have looked at how much time students spend reading textbooks, and when they read. Berry et al. (2010) studied pre-class reading habits of undergraduate students enrolled in finance courses across three different universities. They found that 18% of students report not reading the textbook at all, and approximately 92% of students report spending 3 h or less per week reading. Almost half the students (43%) report reading the textbook for less than an hour a week (Berry et al., 2010). The authors also polled the students to find out when they read. Despite instructors’ recommendation to read before class, very few students actually do so: just 18% of students report that they frequently read before class; 53% report never or rarely reading the textbook before class (Berry et al., 2010). Podolefsky and Finkelstein (2006) found that only 37% of students report regularly reading the textbook and less than 13% read before class. Instead of reading before class, students reported reading predominately in preparation for exams, to find the answer to a specific question, or to help complete homework (Berry et al., 2010).
There are several reasons why students are not reading before class. Some studies suggest that students do not see the connection between doing well on exams and pre-class reading (Podolefsky and Finkelstein, 2006). To strengthen the connection between pre-class reading and course grades, many instructors have implemented graded, pre-class reading quizzes (Burchfield and Sappington, 2000; Connor-Greene, 2000; Ruscio, 2001; Sappington et al., 2002). “Just in-time teaching” (JITT) is one specific implementation of this way of handling pre-class reading (Novak et al., 1999). With JITT, before class, students are required to answer open-ended questions about the reading online, with one question dedicated to soliciting feedback from students about what aspect of the reading they found most confusing. Instructors can use this feedback to tailor their in-class activities and instruction to the most popular areas of student confusion. Even with grade incentives, however, the rate of pre-class reading compliance is still surprisingly low. Stelzer et al. (2009) reported that even with JITT, 70% of students never or rarely read the textbook before class. Heiner et al. (2014) recorded student pre-class reading compliance in two different classes with a JITT-like implementation of short, targeted readings and associated online reading quizzes (Heiner et al., 2014). They found that 79% of students in one class and 85% of students in the other classes reported reading the pre-class reading assignment every week (or most weeks). While these results are promising, this study (as well as the other mentioned studies on pre-class reading) relied on student-reported responses; Sappington et al. (2002) found that students’ self-reported reading compliance is often distorted and invalid.
It is unclear from the literature whether there is a relationship between pre-class reading behavior and in-class exam performance. Podolefsky and Finkelstein (2006) studied the relationship between the frequency with which students report reading and course grades. They conducted this study in three different types of courses: calculus-based physics, algebra-based physics, and conceptual physics. For the calculus and algebra-based courses, they found no significant correlation between course grade and how much students reported reading. For the conceptual course, they found a moderate correlation. Smith and Jacobs (2003) looked at the correlation between time spent reading and course grade for chemistry students and also found no correlation between time spent reading (based on student self-reported data) and course grades. Heiner et al. (2014) found a statistically significant positive correlation between students’ exam performance and the frequency with which students completed the online reading quiz. Because much of the research is based on students’ self-reported data and because of the lack of consensus about the relationship between pre-class reading and grades, we set out to systematically study this relationship.
The research questions we address are:
(1) What are students’ pre-class reading habits on a social learning platform?
(2) Which pre-class reading behaviors are predictive of student in-class exam performance?
(3) What is the efficacy of the platform in promoting student learning?
Theoretical Framework
It is generally accepted that students understand material better after discussing it with others (Bonwell and Eison, 1991; Sorcinelli, 1991). From the social constructivism perspective, students learn through the process of sharing experiences and building knowledge and understanding through discussion (Vygotsky, 1978). Online learning communities are virtual places that combine learning and community together (Downes, 1999) and provide environments for learners to collaboratively build knowledge. Collaborative learning settings provide students a space to verbalize their thinking, build understanding, and solve problems together (Webb et al., 1995; Crouch and Mazur, 2001).
Online discussion forums have been used successfully as tools to facilitate social interactions and exchanges of knowledge between learners (Rovai, 2002; Bradshaw and Hinton, 2004; Tallent-Runnels et al., 2006). The social constructive theory of learning with technology emphasizes that successful learning requires continuous conversation between learners as well as between instructors and learners (Brown and Campione, 1996). As a result, when designing online learning strategies, educators should create social environments with a high degree of interactivity (Maor and Volet, 2007). The asynchronous nature of online discussion forums allows for discussion between learners and between learners and instructors at any time of day or night, and this is a major advantage over other forms of learning environments (Nandi et al., 2009).
Beyond online discussion forums, collaborative annotation systems have recently been developed and used in education as social learning communities. Online annotation systems are computer-mediated communication tools that allow groups of people to collaboratively read and annotate material online. Many studies have shown that online annotation systems increase student learning across many different educational settings (Quade, 1996; Cadiz et al., 2000; Nokelainen et al., 2003; Hwang and Wang, 2004; Marshall and Brush, 2004; Ahren, 2005; Gupta et al., 2008; Robert, 2009; Su et al., 2010).
Perusall: Social Learning Platform for Reading and Annotating
Perusall is an online, social learning platform designed to promote high pre-class reading compliance, engagement, and conceptual understanding. The instructor creates an online course on Perusall, adopting electronic versions of textbooks from publishers or uploading articles or documents, and then creates reading assignments. Students asynchronously annotate the assigned reading by posting (or replying to) comments or questions in a chat-like fashion.
An instructor view of the course home page is shown in Figure 1. The instructor uploads the reading material to the left-hand side of the page (under Documents) and then creates specific reading assignments from these documents which appear in the right panel.
Figure 2 shows what a student sees after opening a reading assignment and highlighting a specific passage on a page in the assignment. A conversation window opens on the right where the student can ask a question or make a comment.
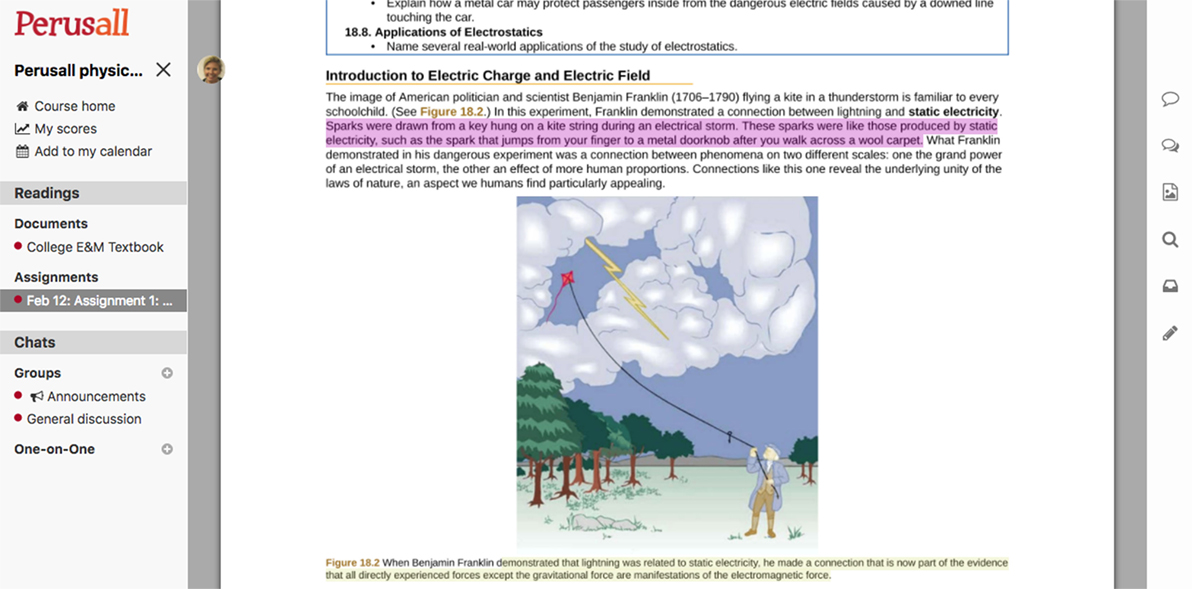
Figure 2. Page of a reading assignment in Perusall. (Note: the depicted individual provided written informed consent for the publication of their identifiable image, the image of the textbook book is from OpenStax, University Physics, Volume 1. “Download for free at https://openstax.org/details/books/university-physics-volume-1,” no further permission is required from the copyright holders for the reproduction of this material.)
Figure 3 shows a page that has been highlighted and annotated by students. When a student clicks on a specific highlight that highlight turns purple, and the conversation window for that highlight opens on the right.
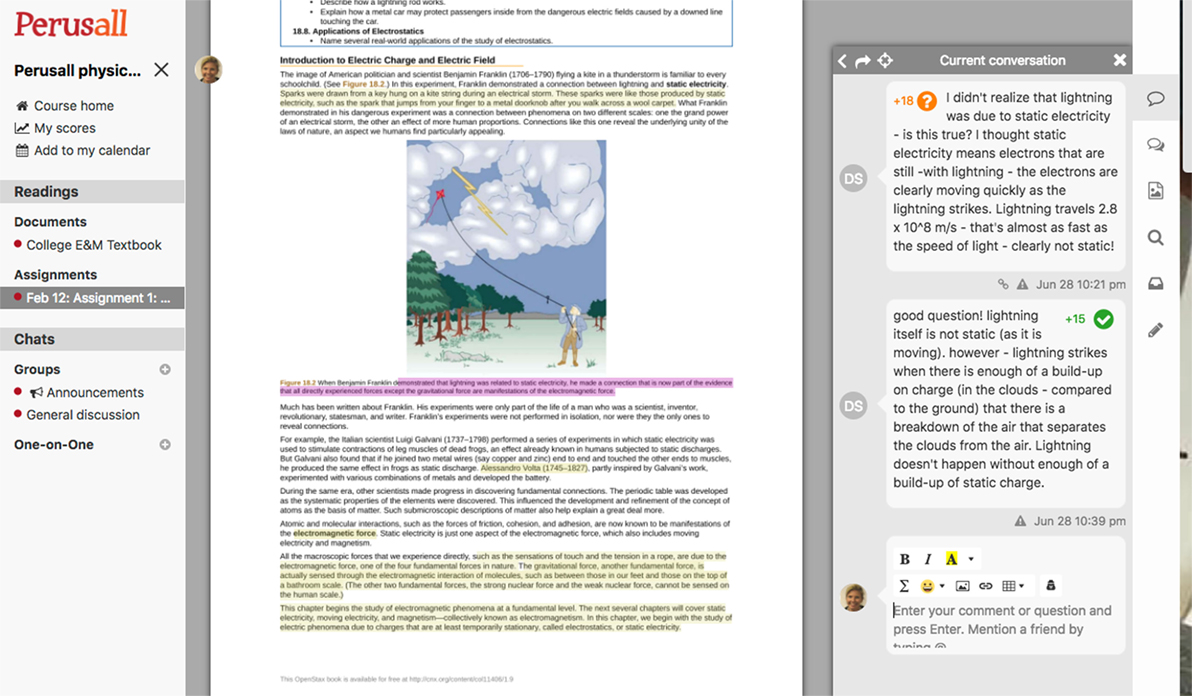
Figure 3. Reading assignment in Perusall showing student highlights and annotations (note: the depicted individual provided written informed consent for the publication of their identifiable image, the image of the textbook book is from OpenStax, University Physics, Volume 1. “Download for free at https://openstax.org/details/books/university-physics-volume-1,” no further permission is required from the copyright holders for the reproduction of this material).
When a student asks a question about a specific passage, it is automatically flagged with an orange question mark, as shown in Figure 3. Other students can respond in an asynchronous conversation.
Perusall also has an integrated assessment tool that provides both students and instructors with constant feedback on how students are engaging with the reading assignments. Finally, Perusall has a built-in tool for instructors called the Confusion Report. This report automatically summarizes the top areas of student confusion for instructors so that they can prepare class material that is targeted specifically to the content that students are struggling with the most.
Social Features
In addition to the basic highlighting and annotating functions, Perusall has a number of additional features designed to turn the online reading assignment into a social experience to encourage students to engage with the material and with fellow classmates outside of class. Several features of the software are designed to promote the social aspect of the software.
Sectioning
If the class exceeds 20 students (or another threshold set by the instructor), the software automatically partitions students in the class into groups that function like “virtual class sections.” Students can only interact with and see annotations posted by others in their group (as well as any annotations posted by the instructor). This allows students to become more familiar with the other students in their group, and this familiarity helps promote more online interaction. Our prior work demonstrated that when the size of the group is too large, the overall quality of students’ annotations decreases (Miller et al., 2016), so these smaller groups prevent students from becoming overwhelmed by an excessively large number annotations and helps keep the overall quality of the interactions high.
Avatars
The avatars of other students and instructors who are viewing the same assignment at the same time appear in the top left hand corner of the screen (Figure 2). Being able to see classmates (and instructors) reading the assignment at the same time increases the social connectivity of the reading experience and encourages students to engage more with the reading (through the software).
Upvoting
Students can provide feedback on the annotations made by other students in their section by “upvoting” annotations. There are two types of upvoting in Perusall. When students would like to know the answer to a question posed by another student, they can indicate this by clicking on the orange question mark. For example, Figure 4 shows that three students clicked on the orange question mark button for that question, indicating that they too would like to know the answer. When instructors review questions in Perusall, they can pay particular attention to the questions that have been upvoted by other students.

Figure 4. Upvoting of questions in Perusall (note: the depicted individual provided written informed consent for the publication of their identifiable image).
When a student provides a particularly helpful explanation, other students can indicate this by clicking on the green checkmark. In Figure 5, five students found the explanation to the initial question to be helpful to their understanding. When students upvote other students’ explanations, it helps other students find explanations that are particularly helpful to their conceptual understanding of the reading. Both of these upvoting features are designed to increase and encourage the social component of the online reading assignments and foster a sense of community within the groups.
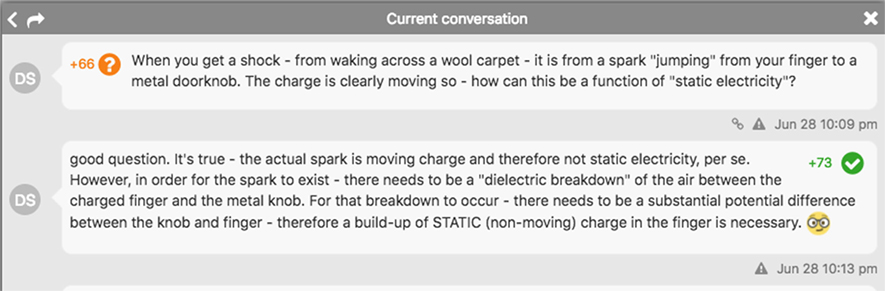
Figure 5. Upvoting of explanations in Perusall (note: the depicted individuals provided written informed consent for the publication of their identifiable images).
Email Notifications
Finally, Perusall has an email notification feature that is designed to encourage the social interaction aspect of the software even when students are not logged into Perusall by letting them know when a classmate has responded to a question or comment they have made (or have clicked the question mark button for). Through the notification, Perusall encourages students to continue their conversation about the reading. Figure 6 shows an example of an email notification that a student receives when a classmate responds to his/her question. The notification encourages students to re-engage with the reading assignment by viewing the conversation and/or letting the responder know whether the response was helpful to their understanding. Students can reply to the email inside their email client, and the reply is appended directly to the conversation in Perusall, as if the student had been online.
Assessment
Perusall has an integrated assessment tool, which automatically evaluates students’ participation in the reading assignment and populates an integrated gradebook (Figure 7).
The grading algorithm uses four criteria to evaluate a students’ collection of annotations for any given reading assignment—timeliness, quantity, quality, and distribution—and students receive an overall score based on all four of these criteria. The grading algorithm uses machine learning to drive desirable student behavior: timely, thorough, and complete reading of the text, with annotations that demonstrate thoughtful interpretation of the subject matter. Students receive a score based on how closely their overall reading and annotating behavior matches behavior that is predictive of success in the classroom.
Instructor Tools
Besides the gradebook and individual reading assignment feedback, which provides important assessment information to both students and instructors, Perusall also assists instructors in identifying from the body of annotations the top areas of confusion so they can prepare class material that is targeted specifically at addressing these areas. To this end Perusall automatically mines questions that students are asking about a particular reading assignment and, using a topic modeling algorithm, groups questions into three to four conceptual areas of confusion. Figure 8 shows an example of a confusion report generated for a specific reading assignment. The philosophy behind the confusion report is based on Just-in-Time-Teaching (Novak, 2011), which uses feedback from work that students do at home (like pre-class reading assignments) to inform what is done in the classroom.
Research Methods
Participants
The participants in this study were undergraduate students enrolled in an introductory physics course. We collected reading assignment data and exam data from two semesters of the course when Perusall was used [spring of 2015 (S15) and fall of 2016 (F16)]. In S15, there were 74 students and in F16 there were 79 students. Students in the S15 course were not the same students as in the F16 course. Due to the fact that the event-tracking feature of the software was not yet developed in S15, most of the analysis focuses on the F16 cohort. As a point of comparison, we also collected exam performance data during the two previous semesters of the course when a different social annotation platform was being used [spring of 2014 (S14) and fall of 2015 (F15)]. There were 72 students enrolled in the S14 course and 75 students enrolled in the F15 course. The four student populations were very similar in composition. Student populations were comprised of 48–50% premedical students and 50–52% engineering students. All four groups were 53–55% female and consisted of equal ratios of students in their sophomore, junior, and senior years of college. The four groups had a similar level of incoming physics background knowledge, as measured by the average score on the physics conceptual survey administered at the beginning of each semester [Force Concept Inventory (Hestenes et al., 1992) for the fall courses and the Conceptual Survey on Electricity and Magnetism (Maloney et al., 2001) for the spring courses].
Setting
We conducted this study in the School of Engineering and Applied Sciences at Harvard University in an introductory physics course called Applied Physics 50 (AP50). AP50 is a calculus-based physics course designed for undergraduate engineering students. It is split into two courses; AP50A, a mechanics course taught in the fall, and AP50B, an electricity and magnetism course taught in the spring.
The instructor was the same for all four semesters and the same pedagogy was used each semester. AP50 met twice weekly and each class was 3-h long. In this course, all lectures were replaced by reading assignments in Perusall and class time was entirely devoted to active learning. The pedagogy was based on features from both Project-Based Learning (Blumenfeld et al., 1991) and Team-Based Learning (Michaelsen et al., 2002). Students worked in small groups for all aspects of the course, including assessments. There were six different types of in-class activities, each of which designed to help students master the relevant physics and get started on the projects, which were the focal point of the course.
As there were no lectures, students were expected to read the textbook on Perusall. By midnight, the night before each class, students were required to complete the pre-class reading assignment by highlighting and annotating an assigned chapter of the textbook, the content of which was the focus of the activities the following day in class. As the class met twice a week, there were typically two chapter-long reading assignments per week. Over the course of each semester there were 17 assigned chapters, with each chapter containing 34 pages on average. To receive full credit for each reading assignment, students needed to enter at least 7 timely and thoughtful annotations per chapter.
Procedure
To evaluate the efficacy of Perusall and to study how and when students were using the software, we did three different types of analyses. We first extracted, from Perusall, a number of metrics that describe student reading behavior: the amount of time students spent reading, how long before each class students logged on to Perusall, and how often they returned to the same reading assignment. We calculated student averages, per reading assignment, for each of these metrics and summarized these (in Figures 9–13) as a way of describing how much and when, relative to class, students were reading. Second, we used the statistical software, STATA, to calculate the correlations between specific reading metrics and student exam performance so that we could determine which (if any) types of reading behaviors are predictive of exam performance. Based on these correlations, we used STATA to develop linear regression models to predict exam performance using reading behavior metrics (controlling for physics background knowledge). Third, to study the efficacy of the software in promoting student learning, we conducted a comparative study between two different types of social annotation software platforms. We compared exam performance during two semesters of AP50 when Perusall was used (S15, F16) to performance on the same exams during two other semesters (S14, F15) when a different social annotation platform was used. The exams in each of the two fall semesters (F15 and F16) were identical as were the exams in each of the two spring semesters (S14 and S15). To ensure that the students’ incoming physics knowledge was the same between the two fall populations and the two spring populations, we used STATA to do a two-sample, t-test for equal means (Snedecor and Cochran, 1989).
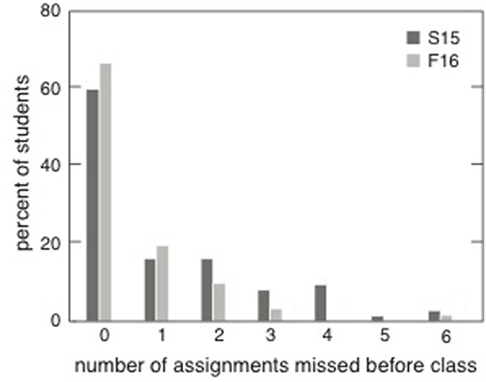
Figure 9. Histogram of the number of assignments students fail to complete before class, in each semester.
This study was carried out in accordance with the recommendations of the Institutional Review Board (IRB) at Harvard University, Committee on the Use of Human Subjects. The IRB classified this study as “minimal risk” and, therefore, exempt from requiring written consent from the participants.
Results
Students’ Pre-Class Behavior on Perusall
Figure 9 shows the extent to which students complete pre-class reading assignments over the two semesters that Perusall was being used. In each semester, approximately 60% of students completed every one of the 17 reading assignments. Figure 9 shows that in S15, approximately 90% of students completed all but a couple of reading assignments; in F16, 95% of students completed all but a couple of reading assignments.
Perusall allows us to collect data on how much time students spend on each individual page of a reading assignment. Using these data, we can determine when a student makes it all the way through the assignment. We define a page as “read” when the time spent on that page is longer than 10 s and less than 20 min. We define a student as having completed an assignment by dividing, for each assignment, the number of pages that were read by the total number of pages in the assignment. Based on this metric, we find that 80% of students make it through at least 95% of the reading and that an additional 10% of students make it through 80% of the reading (Figure 10).
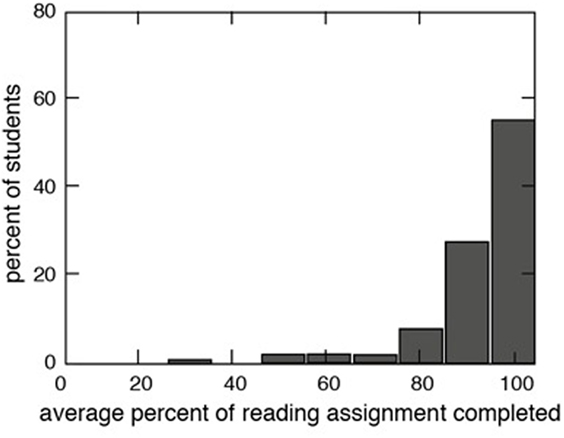
Figure 10. Histogram of the average percent of the reading assignment students complete before class.
Using the same data we find that, on average, students spend 3 h and 20 min per week reading on Perusall (Figure 11).
Figure 12 shows the average number of individual “sessions” students take to complete their reading assignments. We define a session as any cumulative pages read for longer than 10 min with at least 2 h since the previous reading session. On average, students divide their reading of each assignment in seven different sessions.
Figure 13 shows the average number of annotations students enter per assignment. Over the course of the semester, students wrote a total of 16,066 annotations on Perusall. On average students make 13.3 annotations per assignment—nearly twice the number that the system recommends.
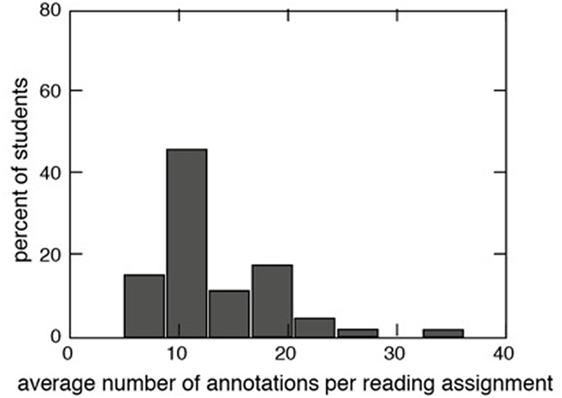
Figure 13. Histogram of average number of annotations entered by students per assignment on Perusall.
Relationship between Student Reading Behavior and In-Class Performance
To study the relationship between reading behavior and in-class performance, we built a series of linear regression models predicting students’ exam performance (averaged over the five exams during the semester) from the reading and annotating metrics previously discussed. These models are presented in Table 1. We control for incoming physics background by including students’ pre-semester score on the Force Concept Inventory (Hestenes et al., 1992) and the Conceptual Survey on Electricity and Magnetism (Maloney et al., 2001). We find that students who break up their reading into more sessions do better on in-class exams than students who read in fewer sessions, even when controlling for pre-course physics knowledge and the amount of time students spend reading.
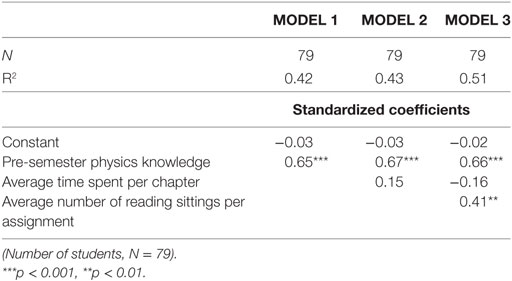
Table 1. Standardized coefficients for linear regression models predicting average exam performance using the average time students spend reading per chapter and the average number of sessions students break their reading up into as predictor variables and controlling for pre-class physics knowledge (pre-semester FCI).
Model 1 shows that we can predict 42% of the variability in students’ average exam performance using only their score on the pre-semester Force Concept Inventory. If we add the average amount of time students spend reading (model 2) we can predict marginally more (43%) of the variability in exam performance although this difference is not significant. When we add to the model the average number of sessions the students use to complete the reading, we find we can predict almost 10% more of the variability in student exam performance (model 3). Increasing the number of sessions a student completes the reading in by one SD increases average student exam performance by 0.41 of a SD (p < 0.01). None of the other reading/annotation metrics are predictive of average student exam performance.
Student In-Class Exam Performance
Finally, we compare two different cohorts of the same course and show that the cohort for which Perusall was used to deliver the pre-class reading assignments did significantly better on the same in-class exams compared to students from the previous year when Perusall was not used.
Figure 14 shows student exam performance on 10 in-class exams administered over 2 years of AP50 (five in the fall semester and five in the spring semester). While the cohort of students were different over the four semesters, Table 2 shows that the four groups of students had the same level of incoming physics knowledge at the beginning of each semester (as measured by the Force Concept Inventory and the Conceptual Survey in Electricity and Magnetism). We conducted two-sample, t-tests to confirm that the performance on these conceptual inventories was the same for the two fall groups and for the two spring groups (p = 0.32 and p = 0.36, respectively).
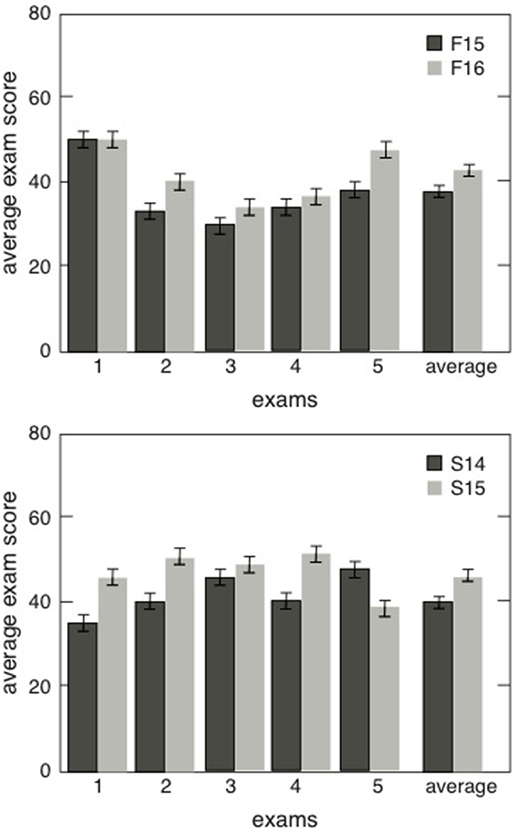
Figure 14. Exam performance comparison between two different cohorts of the same course where the only difference is the use of Perusall as the online learning platform. The error bars represent the SEM.
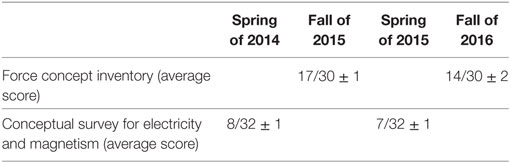
Table 2. Comparison of pre-course conceptual physics survey results between the four semesters of students (S14/F15 when Perusall was not used compared to S15/F16 when Perusall was used).
The only difference in the course between the S14/F15 and S15/F16 was the use of Perusall. During the S14/F15 semesters a simpler annotation tool was used to administer the pre-class reading assignments. This annotation tool lacked many of the social and machine learning features of Perusall. Students in the S15/F16 semesters scored 5–10% better on all but two of the 10 exams compared to the students from the semesters before when Perusall was not being used (p < 0.05). Based on a two-sample t-test, averaging over all five exams in the fall, students in the class that used Perusall scored significantly better than the class that did not use Perusall (p < 0.05). Students in the fall class that did not use Perusall had an average exam score of 38% compared to students in the fall class that used Perusall who had an average exam score of 43% (effect size = 0.34). The same is true when we average over all five exams in the spring—students in the class that used Perusall outperformed the students from the year before (p < 0.05). Students in the spring class that did not use Perusall had an average exam score of 41% compared to students in the spring class that used Perusall who had an average exam score of 45% (effect size = 0.31).
Discussion and Conclusion
This study explores student pre-class reading behavior on Perusall, a social learning platform that allows students to interact and discuss course material online. We find that student completion of reading assignments is substantially higher than what has been reported in the vast majority of the literature. With Perusall, 90–95% of students complete all but a few of the reading assignments before class. For comparison, most of the literature reports that 60–80% of students do not read the textbook before coming to class (Cummings et al., 2002; Clump et al., 2004; Podolefsky and Finkelstein, 2006; Stelzer et al., 2009). One study found that, with a JITT-like implementation of pre-class reading, between 80 and 85% of students completed the reading before class (Heiner et al., 2014), but this study was based on student-reported reading data, which has been shown to be unreliable. Using reading data from Perusall, we find that 80% of students complete 100% of the reading assignment before class. This percentage, too, is considerably higher than what is reported in the literature: Clump et al. (2004) find that students only read on average 28% of the assigned reading before class.
In addition to higher completion of pre-class reading assignment, we also find that, on Perusall, students read for longer than what is reported in the literature. Approximately 92% of students report that they spent 3 h or less per week reading the textbook. On Perusall, students spend, on average, 3 h and 20 min per week reading for this one course.
In studying the relationship between reading behavior and in-class performance, we find that the average time spent reading per chapter alone is not predictive of student exam performance. This is consistent with what has already been reported in the literature (Smith and Jacobs, 2003; Podolefsky and Finkelstein, 2006). However, it should be noted that previous studies on the relationship between time spent reading and exam performance have all been based on student-reported data. Our study uses data obtained directly from the Perusall platform. We do find, however, that students who break the reading up into more reading sittings perform better on in-class exams than students who read in fewer sittings. This is true even when we control for the amount of time students spent reading, and consistent given the spacing effect, a well-known phenomenon in psychology: material is more effectively and easily learned when it is studied over several times spaced out over a longer time span, rather than trying to learn it in a short period of time (Dempster and Farris, 1990).
Finally, we find that students using Perusall perform significantly better on in-class exams than students using a simple annotation tool without some of the social and machine learning features of Perusall. We recognize that this result does not indicate causality and must be interpreted carefully given the fact that other factors could be confounding the results. More research needs to be done to pinpoint exactly why students do significantly better using Perusall. Perusall has many features that the other platform did not have. For example, with Perusall assessment is built right into the platform and students get regular and timely feedback. In the other platform, assessment was provided separately by the instructors and so students received sporadic and less targeted feedback. Perusall also has many social features (sectioning, avatars, upvoting, email notifications) that are designed to improve the interactions between students. Finally, the Confusion Report makes it easier for the instructor to address main areas of student confusion in class, which both affords better targeting of in-class time to student confusion and allows students to better see the connection between pre-class reading assignments and in-class activities.
We have demonstrated the efficacy of Perusall as a social learning platform and have shown that student completion of pre-class reading assignment is substantially higher than what has been reported by other studies. In short, with Perusall we are better able to get students to complete reading assignments, and do so in a way—with spaced repetition—that leads to better outcomes. Perusall, therefore, is a useful tool for delivering content to students outside class and for building an online learning community in which students can discuss course content and develop understanding. This is particularly important in flipped and hybrid courses or any other course that relies on pre-class reading assignments.
Ethics Statement
We were covered for this research by Harvard’s Committee on the Use of Human Subjects (CUHS).
Author Contributions
Several people contributed to the work described in this paper. EM conceived of the basic idea for this work. KM, BL, GK, and EM designed and carried out the study, and KM analyzed the results and wrote the first draft of the paper. All authors contributed to the development of the manuscript.
Conflict of Interest Statement
The authors developed the technology described in this article, mostly at Harvard University. Perusall.com is a commercial product based on this work. The authors are cofounders of Perusall, LLC, the company that runs perusall.com.
References
Ahren, T. C. (2005). Using online annotation software to provide timely feedback in an introductory programming course. Paper Presented at the 35th ASEE/IEEE Frontiers in Education Conference, Indiannapolis, IN. Available at: http://www.icee.usm.edu/icee/conferences/FIEC2005/papers/1696.pdf
Berry, T., Cook, L., Hill, N., and Stevens, K. (2010). An exploratory analysis of textbook usage and study habits: Misperceptions and barriers to success. Coll. Teach. 59, 31–39.
Blumenfeld, P. C., Soloway, E., Marx, R. W., Krajcik, J. S., Guzdial, M., and Palincsar, A. (1991). Motivating project-based learning: sustaining the doing, supporting the learning. Educ. Psychol. 26, 369–398. doi: 10.1080/00461520.1991.9653139
Bonwell, C. C., and Eison, J. A. (1991). Active Learning: Creating Excitement in the Classroom. 1991 ASHE-ERIC Higher Education Reports. Washington, DC: ERIC Clearinghouse on Higher Education.
Bradshaw, J., and Hinton, L. (2004). Benefits of an online discussion list in a traditional distance education course. Turk. Online J. Distance Educ. 5.
Brown, A., and Campione, J. (1996). “Psychological theory and design of innovative learning environments: on procedures principles and systems,” in Innovations in Learning: New Environments for Education, eds L. Schauble, and R. Glaser (Mahwah, NJ: Erlbaum), 289–325.
Burchfield, C. M., and Sappington, J. (2000). Compliance with required reading assignments. Teach. Psychol. 27, 58–60.
Cadiz, J. J., Gupta, A., and Grudin, J. (2000). “Using web annotations for asynchronous collaboration around documents,” in Proceedings of CSCW’00: The 2000 ACM Conference on Computer Supported Cooperative Work (Philadelphia, PA: ACM), 309–318.
Clump, M. A., Bauer, H., and Bradley, C. (2004). The extent to which psychology students read textbooks: a multiple class analysis of reading across the psychology curriculum. J. Instr. Psychol. 31, 227–232.
Connor-Greene, P. A. (2000). Assessing and promoting student learning: blurring the line between teaching and testing. Teach. Psychol. 27, 84–88. doi:10.1207/S15328023TOP2702_01
Crouch, C. H., and Mazur, E. (2001). Peer instruction: ten years of experience and results. Am. J. Phys. 69, 970–997. doi:10.1119/1.1374249
Cummings, K., French, T., and Cooney, P. J. (2002). “Student textbook use in introductory physics,” in Proceedings of PERC’02: In Physics Education Research Conference, eds S. Franklin, K. Cummings, and J. Marx (Boise, ID), 7–8.
Dempster, F. N., and Farris, R. (1990). The spacing effect: research and practice. J. Res. Dev. Educ. 23, 97–101.
Dobson, J. L. (2008). The use of formative online quizzes to enhance class preparation and scores on summative exams. Adv. Physiol. Educ. 32, 297–302. doi:10.1152/advan.90162.2008
Downes, S. (1999). Creating an Online Learning Community [PowerPoint Slides]. Edmonton: Virtual School Symposium. Available at: https://www.slideshare.net/Downes/creating-an-online-learning-community
Gupta, S., Condit, C., and Gupta, A. (2008). Graphitti: an annotation management system for heterogeneous objects. Paper Presented at IEEE 24th International Conference on Data Engineering, Cancun, Mexico, 1568–1571.
Heiner, C. E., Banet, A. I., and Wieman, C. (2014). Preparing students for class: How to get 80% of students reading the textbook before class. Am. J. Phys. 82, 989–996.
Herreid, C., and Schiller, N. (2013). Case studies and the flipped classroom. J. Coll. Sci. Teach. 42, 62–66.
Hestenes, D., Wells, M., and Swackhamer, G. (1992). Force concept inventory. Phys. Teach. 30, 141–157. doi:10.1119/1.2343497
Hwang, W. Y., and Wang, C. Y. (2004). “A study on application of annotation system in web-based materials,” in Proceedings of GCCCE’ 04: the 8th Global Chinese Conference on Computers in Education, Hong Kong.
Johnson, B. C., and Kiviniemi, M. T. (2009). The effect of online chapter quizzes on exam performance in an undergraduate social psychology course. Teach. Psychol. 36, 33–37. doi:10.1080/00986280802528972
Karp, D. A., and Yoels, W. C. (1976). The college classroom: some observations on the meanings of student participation. Sociol. Soc. Res. 60, 421–439.
Maloney, D. P., O’Kuma, T. L., Hieggelke, C. J., and Van Heuvelen, A. (2001). Surveying students’ conceptual knowledge of electricity and magnetism. Am. J. Phy. 69, S12–S23. doi:10.1119/1.1371296
Maor, D., and Volet, S. (2007). Interactivity in professional learning: a review of research based studies. Aust. J. Educ. Technol. 23, 227–247. doi:10.14742/ajet.1268
Marcell, M. (2008). Effectiveness of regular online quizzing in increasing class participation and preparation. Int. J. Scholarsh. Teach. Learn. 2, 7. doi:10.20429/ijsotl.2008.020107
Marshall, C. C., and Brush, A. J. B. (2004). “Exploring the relationship between personal and public annotations,” in Proceedings of JCDL’04: The 2004 ACE/IEEE Conference on Digital Libraries (Tucson, AZ), 349–357.
Michaelsen, L. K., Knight, A. B., and Fink, L. D. (eds) (2002). Team-Based Learning: A Transformative Use of Small Groups. Westport, CT: Greenwood Publishing Group.
Miller, K., Zyto, S., Karger, D., Yoo, J., and Mazur, E. (2016). Analysis of student engagement in an online annotation system in the context of a flipped introductory physics class. Phys. Rev. Phys. Educ. Res. 12, 020143. doi:10.1103/PhysRevPhysEducRes.12.020143
Nandi, D., Chang, S., and Balbo, S. (2009). “A conceptual framework for assessing interaction quality in online discussion forums,” in Same Places, Different Spaces. Proceedings Ascilite Auckland 2009.
Narloch, R., Garbin, C. P., and Turnage, K. D. (2006). Benefits of prelecture quizzes. Teach. Psychol. 33, 109–112. doi:10.1207/s15328023top3302_6
Nokelainen, P., Kurhila, J., Miettinen, M., Floreen, P., and Tirri, H. (2003). “Evaluating the role of a shared document-based annotation tool in learner-centered collaborative learning,” in Proceedings of ICALT’03: the 3rd IEEE International Conference on Advanced Learning Technologies (Athens), 200–203.
Novak, G. M., Patterson, E. T., Gavrin, A. D., and Christian, W. (1999). Just-in-Time Teaching: Blending Active Learning with Web Technology. Upper Saddle River, NJ: Addison-Wesley.
Podolefsky, N., and Finkelstein, N. (2006). The perceived value of college physics textbooks: students and instructors may not see eye to eye. Phys. Teach. 44, 338–342. doi:10.1119/1.2336132
Quade, A. M. (1996). “An assessment of retention and depth of processing associated with note taking using traditional pencil and paper and an on-line notepad during computer-delivered instruction,” in Proceedings of Selected Research and Development Presentations at the 1996 National Convention of the Association for Educational Communications and Technology (Indianapolis, IN), 559–570.
Robert, C. A. (2009). Annotation for knowledge sharing in a collaborative environment. J. Knowl. Manag. 13, 111–119. doi:10.1108/13673270910931206
Rovai, A. P. (2002). Building sense of community at a distance. Int. Rev. Res. Open Distrib. Learn. 3. doi:10.19173/irrodl.v3i1.79
Ruscio, J. (2001). Administering quizzes at random to increase students’ reading. Teach. Psychol. 28, 204–206.
Sappington, J., Kinsey, K., and Munsayac, K. (2002). Two studies of reading compliance among college students. Teach. Psychol. 29, 272–274. doi:10.1207/S15328023TOP2904_02
Schwartz, D. L., and Bransford, J. D. (1998). A time for telling. Cogn. Instr. 16, 475–5223. doi:10.1207/s1532690xci1604_4
Smith, B. D., and Jacobs, D. C. (2003). TextRev: a window into how general and organic chemistry students use textbook resources. J. Chem. Educ. 80, 99. doi:10.1021/ed080p99
Snedecor, G. W., and Cochran, W. G. (1989). Analysis of Variance: The Random Effects Model. Statistical Methods. Ames, IA: Iowa State University Press, 237–252.
Sorcinelli, M. D. (1991). Research findings on the seven principles. New Directions for Teaching and Learning. 1991, 13–25. doi:10.1002/tl.37219914704
Stelzer, T., Gladding, G., Mestre, J. P., and Brookes, D. T. (2009). Comparing the efficacy of multimedia modules with traditional textbooks for learning introductory physics content. Am. J. Phys. 77, 184–190. doi:10.1119/1.3028204
Su, A. Y. S., Yang, S. H., Hwang, W. Y., and Zhang, J. (2010). A web 2.0-based collaborative annotation system for enhancing knowledge sharing in collaborative learning environments. Comput. Educ. 55, 752–766. doi:10.1016/j.compedu.2010.03.008
Tallent-Runnels, M. K., Thomas, J. A., Lan, W. Y., Cooper, S., Ahern, T. C., Shaw, S. M., et al. (2006). Teaching courses online: a review of the research. Rev. Educ. Res. 76, 93–135. doi:10.3102/00346543076001093
Vygotsky, L. S. (1978). Mind in Society: The Development of Higher Psychological Processes. Cambridge, MA: Cambridge University Press.
Keywords: digital education, flipped classroom, educational software, pre-class reading, physics education research
Citation: Miller K, Lukoff B, King G and Mazur E (2018) Use of a Social Annotation Platform for Pre-Class Reading Assignments in a Flipped Introductory Physics Class. Front. Educ. 3:8. doi: 10.3389/feduc.2018.00008
Received: 29 September 2017; Accepted: 19 January 2018;
Published: 07 March 2018
Edited by:
Elizabeth S. Charles, Dawson College, CanadaReviewed by:
Liisa Ilomäki, University of Helsinki, FinlandMichael Dugdale, John Abbott College, Canada
Copyright: © 2018 Miller, Lukoff, King and Mazur. This is an open-access article distributed under the terms of the Creative Commons Attribution License (CC BY). The use, distribution or reproduction in other forums is permitted, provided the original author(s) and the copyright owner are credited and that the original publication in this journal is cited, in accordance with accepted academic practice. No use, distribution or reproduction is permitted which does not comply with these terms.
*Correspondence: Kelly Miller, kmiller@seas.harvard.edu