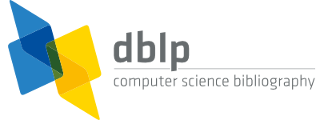


default search action
OpML 2019: Santa Clara, CA, USA
- Bharath Ramsundar, Nisha Talagala:
2019 USENIX Conference on Operational Machine Learning, OpML 2019, Santa Clara, CA, USA, May 20, 2019. USENIX Association 2019
Production Experiences and Learnings
- Rajagopal Ananthanarayanan, Peter Brandt, Manasi Joshi, Maheswaran Sathiamoorthy:
Opportunities and Challenges Of Machine Learning Accelerators In Production. 1-3 - Minjia Zhang, Samyam Rajbhandari, Wenhan Wang, Elton Zheng, Olatunji Ruwase, Jeff Rasley, Jason Li, Junhua Wang, Yuxiong He:
Accelerating Large Scale Deep Learning Inference through DeepCPU at Microsoft. 5-7 - Junsung Lim, Hoejoo Lee, Youngmin Won, Hunje Yeon:
MLOp Lifecycle Scheme for Vision-based Inspection Process in Manufacturing. 9-11 - Ankit Arun, Ignacio Arnaldo:
Shooting the moving target: machine learning in cybersecurity. 13-14 - Jonathan Soifer, Jason Li, Mingqin Li, Jeffrey Zhu, Yingnan Li, Yuxiong He, Elton Zheng, Adi Oltean, Maya Mosyak, Chris Barnes, Thomas Liu, Junhua Wang:
Deep Learning Inference Service at Microsoft. 15-17
Handling Heterogeneity, Distribution, and Scale
- Zheng Chai, Hannan Fayyaz, Zeshan Fayyaz, Ali Anwar, Yi Zhou, Nathalie Baracaldo, Heiko Ludwig, Yue Cheng:
Towards Taming the Resource and Data Heterogeneity in Federated Learning. 19-21
Measuring and Diagnosing Production ML
- Sindhu Ghanta, Sriram Subramanian, Lior Khermosh, Harshil Shah, Yakov Goldberg, Swaminathan Sundararaman, Drew S. Roselli, Nisha Talagala:
MPP: Model Performance Predictor. 23-25
Optimizing and Tuning
- Hidehito Yabuuchi, Daisuke Taniwaki, Shingo Omura:
Low-latency Job Scheduling with Preemption for the Development of Deep Learning. 27-30 - Sayed Hadi Hashemi, Paul Rausch, Benjamin Rabe, Kuan-Yen Chou, Simeng Liu, Volodymyr V. Kindratenko, Roy H. Campbell:
tensorflow-tracing: A Performance Tuning Framework for Production. 31-33 - Ken Yocum, Sean Rowan, Jonathan Lunt, Theodore M. Wong:
Disdat: Bundle Data Management for Machine Learning Pipelines. 35-37 - Anthony Hsu, Keqiu Hu, Jonathan Hung, Arun Suresh, Zhe Zhang:
TonY: An Orchestrator for Distributed Machine Learning Jobs. 39-41 - Md Shahriar Iqbal, Lars Kotthoff, Pooyan Jamshidi:
Transfer Learning for Performance Modeling of Deep Neural Network Systems. 43-46
Solutions and Platforms
- Saman Biookaghazadeh, Yitao Chen, Kaiqi Zhao, Ming Zhao:
KnowledgeNet: Disaggregated and Distributed Training and Serving of Deep Neural Networks. 47-49 - Denis Baylor, Kevin Haas, Konstantinos Katsiapis, Sammy Leong, Rose Liu, Clemens Mewald, Hui Miao, Neoklis Polyzotis, Mitchell Trott, Martin Zinkevich:
Continuous Training for Production ML in the TensorFlow Extended (TFX) Platform. 51-53 - Jinan Zhou, Andrey Velichkevich, Kirill Prosvirov, Anubhav Garg, Yuji Oshima, Debo Dutta:
Katib: A Distributed General AutoML Platform on Kubernetes. 55-57 - Anirban Bhattacharjee, Yogesh D. Barve, Shweta Khare, Shunxing Bao, Aniruddha Gokhale, Thomas Damiano:
Stratum: A Serverless Framework for the Lifecycle Management of Machine Learning-based Data Analytics Tasks. 59-61

manage site settings
To protect your privacy, all features that rely on external API calls from your browser are turned off by default. You need to opt-in for them to become active. All settings here will be stored as cookies with your web browser. For more information see our F.A.Q.