Gene Ontology Curation of Neuroinflammation Biology Improves the Interpretation of Alzheimer's Disease Gene Expression Data
- PMID: 32417785
- PMCID: PMC7369085
- DOI: 10.3233/JAD-200207
Gene Ontology Curation of Neuroinflammation Biology Improves the Interpretation of Alzheimer's Disease Gene Expression Data
Abstract
Background: Gene Ontology (GO) is a major bioinformatic resource used for analysis of large biomedical datasets, for example from genome-wide association studies, applied universally across biological fields, including Alzheimer's disease (AD) research.
Objective: We aim to demonstrate the applicability of GO for interpretation of AD datasets to improve the understanding of the underlying molecular disease mechanisms, including the involvement of inflammatory pathways and dysregulated microRNAs (miRs).
Methods: We have undertaken a systematic full article GO annotation approach focused on microglial proteins implicated in AD and the miRs regulating their expression. PANTHER was used for enrichment analysis of previously published AD data. Cytoscape was used for visualizing and analyzing miR-target interactions captured from published experimental evidence.
Results: We contributed 3,084 new annotations for 494 entities, i.e., on average six new annotations per entity. This included a total of 1,352 annotations for 40 prioritized microglial proteins implicated in AD and 66 miRs regulating their expression, yielding an average of twelve annotations per prioritized entity. The updated GO resource was then used to re-analyze previously published data. The re-analysis showed novel processes associated with AD-related genes, not identified in the original study, such as 'gliogenesis', 'regulation of neuron projection development', or 'response to cytokine', demonstrating enhanced applicability of GO for neuroscience research.
Conclusions: This study highlights ongoing development of the neurobiological aspects of GO and demonstrates the value of biocuration activities in the area, thus helping to delineate the molecular bases of AD to aid the development of diagnostic tools and treatments.
Keywords: Alzheimer’s disease; Cytoscape network analysis; Gene Ontology; PANTHER; microglia; neuroinflammation.
Conflict of interest statement
Authors’ disclosures available online (
Figures
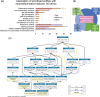
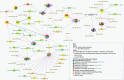
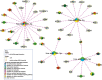
Similar articles
-
Gene Ontology curation of the blood-brain barrier to improve the analysis of Alzheimer's and other neurological diseases.Database (Oxford). 2021 Oct 26;2021:baab067. doi: 10.1093/database/baab067. Database (Oxford). 2021. PMID: 34697638 Free PMC article.
-
Best Practices in Manual Annotation with the Gene Ontology.Methods Mol Biol. 2017;1446:41-54. doi: 10.1007/978-1-4939-3743-1_4. Methods Mol Biol. 2017. PMID: 27812934
-
Gene Ontology: A Resource for Analysis and Interpretation of Alzheimer’s Disease Data.In: Wisniewski T, editor. Alzheimer’s Disease [Internet]. Brisbane (AU): Codon Publications; 2019 Dec 20. Chapter 2. In: Wisniewski T, editor. Alzheimer’s Disease [Internet]. Brisbane (AU): Codon Publications; 2019 Dec 20. Chapter 2. PMID: 31895510 Free Books & Documents. Review.
-
Integrated analysis of miRNA and mRNA expression in the blood of patients with Alzheimer's disease.Mol Med Rep. 2020 Aug;22(2):1053-1062. doi: 10.3892/mmr.2020.11162. Epub 2020 May 20. Mol Med Rep. 2020. PMID: 32468026
-
How Does the Scientific Community Contribute to Gene Ontology?Methods Mol Biol. 2017;1446:85-93. doi: 10.1007/978-1-4939-3743-1_7. Methods Mol Biol. 2017. PMID: 27812937 Review.
Cited by
-
Representation of non-coding RNA-mediated regulation of gene expression using the Gene Ontology.RNA Biol. 2024 Jan;21(1):36-48. doi: 10.1080/15476286.2024.2408523. Epub 2024 Oct 7. RNA Biol. 2024. PMID: 39374113 Free PMC article.
-
Exploring automatic inconsistency detection for literature-based gene ontology annotation.Bioinformatics. 2022 Jun 24;38(Suppl 1):i273-i281. doi: 10.1093/bioinformatics/btac230. Bioinformatics. 2022. PMID: 35758780 Free PMC article.
-
Genome-Wide Association Study of Body Weight Traits in Inner Mongolia Cashmere Goats.Front Vet Sci. 2021 Dec 1;8:752746. doi: 10.3389/fvets.2021.752746. eCollection 2021. Front Vet Sci. 2021. PMID: 34926636 Free PMC article.
-
Gene Ontology curation of the blood-brain barrier to improve the analysis of Alzheimer's and other neurological diseases.Database (Oxford). 2021 Oct 26;2021:baab067. doi: 10.1093/database/baab067. Database (Oxford). 2021. PMID: 34697638 Free PMC article.
-
UniProt: the universal protein knowledgebase in 2021.Nucleic Acids Res. 2021 Jan 8;49(D1):D480-D489. doi: 10.1093/nar/gkaa1100. Nucleic Acids Res. 2021. PMID: 33237286 Free PMC article.
References
-
- Lane CA, Hardy J, Schott JM (2018) Alzheimer’s disease. Eur J Neurol 25, 59–70. - PubMed
-
- Griffiths HH, Morten IJ, Hooper NM (2008) Emerging and potential therapies for Alzheimer’s disease. Expert Opin Ther Targets 12, 693–704. - PubMed
-
- Loera-Valencia R, Cedazo-Minguez A, Kenigsberg P, Page G, Duarte A, Giusti P, Zusso M, Robert P, Frisoni GB, Cattaneo A, Zille M, Boltze J, Cartier N, Buee L, Johansson G, Winblad B (2019) Current and emerging avenues for Alzheimer’s disease drug targets.398-437. J Intern Med 286. - PubMed
Publication types
MeSH terms
Grants and funding
LinkOut - more resources
Full Text Sources
Medical