Imaging body-mind crosstalk in young adults
- PMID: 39290876
- PMCID: PMC11407095
- DOI: 10.1016/j.ijchp.2024.100498
Imaging body-mind crosstalk in young adults
Abstract
Objective: There is evidence that complex relationships exist between motor functions, brain structure, and cognitive functions, particularly in the aging population. However, whether such relationships observed in older adults could extend to other age groups (e.g., younger adults) remains to be elucidated. Thus, the current study addressed this gap in the literature by investigating potential associations between motor functions, brain structure, and cognitive functions in a large cohort of young adults.
Methods: In the current study, data from 910 participants (22-35 yr) were retrieved from the Human Connectome Project. Interactions between motor functions (i.e., cardiorespiratory fitness, gait speed, hand dexterity, and handgrip strength), brain structure (i.e., cortical thickness, surface area, and subcortical volumes), and cognitive functions were examined using linear mixed-effects models and mediation analyses. The performance of different machine-learning classifiers to discriminate young adults at three different levels (related to each motor function) was compared.
Results: Cardiorespiratory fitness and hand dexterity were positively associated with fluid and crystallized intelligence in young adults, whereas gait speed and handgrip strength were correlated with specific measures of fluid intelligence (e.g., inhibitory control, flexibility, sustained attention, and spatial orientation; false discovery rate [FDR] corrected, p < 0.05). The relationships between cardiorespiratory fitness and domains of cognitive function were mediated by surface area and cortical volume in regions involved in the default mode, sensorimotor, and limbic networks (FDR corrected, p < 0.05). Associations between handgrip strength and fluid intelligence were mediated by surface area and volume in regions involved in the salience and limbic networks (FDR corrected, p < 0.05). Four machine-learning classifiers with feature importance ranking were built to discriminate young adults with different levels of cardiorespiratory fitness (random forest), gait speed, hand dexterity (support vector machine with the radial kernel), and handgrip strength (artificial neural network).
Conclusions: In summary, similar to observations in older adults, the current study provides empirical evidence (i) that motor functions in young adults are positively related to specific measures of cognitive functions, and (ii) that such relationships are at least partially mediated by distinct brain structures. Furthermore, our analyses suggest that machine-learning classifier has a promising potential to be used as a classification tool and decision support for identifying populations with below-average motor and cognitive functions.
Keywords: Brain structure; Fluid and crystallized intelligence; Machine learning; Motor function; Young adults.
© 2024 The Authors.
Conflict of interest statement
The authors declare that they have no known competing financial interests or personal relationships that could have appeared to influence the work reported in this paper.
Figures
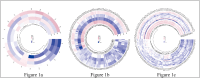
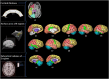
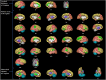
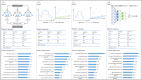
Similar articles
-
Predictive models demonstrate age-dependent association of subcortical volumes and cognitive measures.Hum Brain Mapp. 2023 Feb 1;44(2):801-812. doi: 10.1002/hbm.26100. Epub 2022 Oct 12. Hum Brain Mapp. 2023. PMID: 36222055 Free PMC article.
-
Identification of the brain networks that contribute to the interaction between physical function and working memory: An fMRI investigation with over 1,000 healthy adults.Neuroimage. 2020 Nov 1;221:117152. doi: 10.1016/j.neuroimage.2020.117152. Epub 2020 Jul 12. Neuroimage. 2020. PMID: 32668299
-
Bidirectional associations of high-level cognitive domains with hand motor function and gait speed in high-functioning older adults: A 7-year study.Arch Gerontol Geriatr. 2024 Feb;117:105232. doi: 10.1016/j.archger.2023.105232. Epub 2023 Oct 27. Arch Gerontol Geriatr. 2024. PMID: 37956584
-
Observational Evidence of the Association Between Handgrip Strength, Hand Dexterity, and Cognitive Performance in Community-Dwelling Older Adults: A Systematic Review.J Epidemiol. 2018 Sep 5;28(9):373-381. doi: 10.2188/jea.JE20170041. Epub 2018 Mar 10. J Epidemiol. 2018. PMID: 29526916 Free PMC article. Review.
-
Exercise interventions for cerebral palsy.Cochrane Database Syst Rev. 2017 Jun 11;6(6):CD011660. doi: 10.1002/14651858.CD011660.pub2. Cochrane Database Syst Rev. 2017. PMID: 28602046 Free PMC article. Review.
References
-
- Allaire, J., Chollet, F., Tang, Y., Falbel, D., Van Der Bijl, W., Studer, M., & Allaire, M.J. (2022). Package ‘keras’. R Interface to ‘Keras.
-
- Allali G., Annweiler C., Blumen H.M., Callisaya M.L., De Cock A.M., Kressig R.W., Srikanth V., Steinmetz J.P., Verghese J., Beauchet O. Gait phenotype from mild cognitive impairment to moderate dementia: Results from the GOOD initiative. European Journal of Neurology. 2016;23(3):527–541. - PMC - PubMed
Grants and funding
LinkOut - more resources
Full Text Sources
Miscellaneous